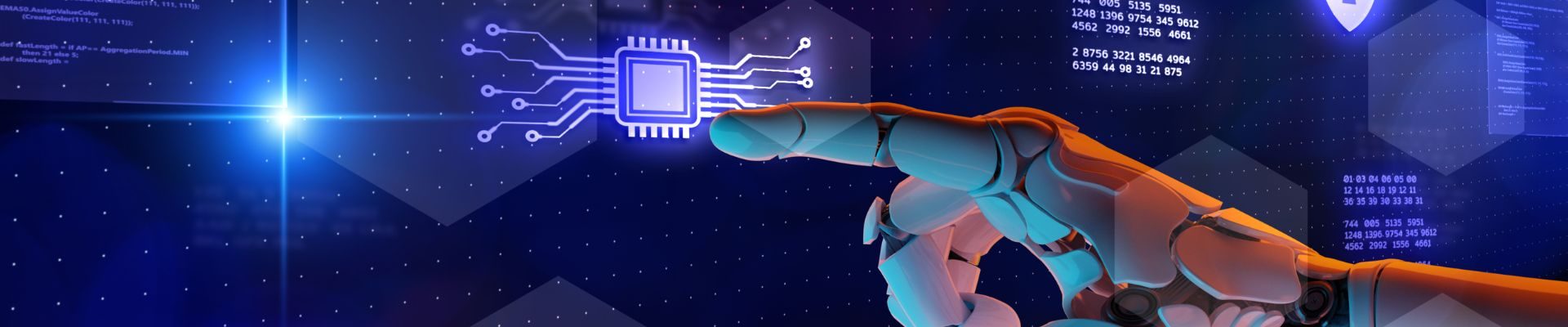
Exploring the World of Generative AI
In recent years, the field of artificial intelligence has witnessed remarkable advancements, particularly in a fascinating area known as generative AI. This technology has the ability to create new content, ranging from images and music to text and even entire stories, mimicking human-like creativity. Let’s delve into the captivating world of generative AI and understand its applications, challenges, and future prospects.
What is Generative AI?
Generative AI refers to a class of machine learning algorithms that aim to generate new data samples resembling a given dataset. Unlike traditional AI models that focus on specific tasks like classification or prediction, generative AI models are designed to understand and mimic patterns in data in order to generate novel outputs.
One of the key techniques used in generative AI is Generative Adversarial Networks (GANs). GANs consist of two neural networks: a generator and a discriminator. The generator is tasked with creating synthetic data samples, while the discriminator’s role is to differentiate between real and generated data. Through an adversarial training process, both networks improve iteratively, resulting in highly realistic generated outputs.
Applications of Generative AI
Generative AI has found diverse applications across various domains:
Art and Creativity: Generative AI models can autonomously create artwork, music compositions, and even literature. This technology enables artists and creators to explore new ideas and styles, pushing the boundaries of human creativity.
Content Generation: In media and entertainment, generative AI is used to generate personalized content such as movie recommendations, news articles, and virtual characters for games.
Data Augmentation: Generative models can be used to synthesize new data samples for training machine learning models. This is particularly useful in scenarios where labeled data is limited.
Drug Discovery: In pharmaceutical research, generative AI is employed to design novel drug molecules with specific properties, potentially accelerating the drug discovery process.
Image Synthesis and Editing: Generative models like StyleGAN are capable of generating high-resolution images of human faces, enabling applications in virtual fashion, face generation, and more.
Challenges and Ethical Considerations
While generative AI holds immense promise, it also presents several challenges:
Bias and Fairness: Generative models trained on biased datasets may produce biased outputs, raising concerns about fairness and inclusivity.
Ethical Use: The use of AI-generated content raises ethical questions, especially in areas like deepfakes and misinformation.
Robustness and Control: Ensuring the robustness and control of generative models is crucial to prevent unintended consequences.
Future Outlook
The future of generative AI looks promising with ongoing research and development. Key areas of advancement include:
Improved Realism: Advancements in GANs and other generative models will lead to more realistic and diverse outputs.
Interdisciplinary Applications: Generative AI will continue to impact diverse fields such as healthcare, design, and education.
Ethical Guidelines: Efforts are underway to establish ethical guidelines and regulations for the responsible use of generative AI.
In conclusion, generative AI represents a paradigm shift in the capabilities of artificial intelligence, unlocking new avenues for creativity and innovation. While challenges remain, the potential benefits of generative AI are vast, promising transformative applications across industries and domains.
Posts
Contact
- contact@devfanclub.com
- +91 9209997139
5 Responses